In today’s digital age, events have become a crucial aspect of businesses and organizations. From conferences, trade shows, product launches, to webinars and virtual events, companies are constantly looking for ways to engage with their target audience and generate leads. But hosting an event is not just about the day itself; it also involves a lot of planning, promotion, and post-event analysis.
Post-event analysis is essential for understanding the success of an event, identifying areas for improvement, and informing future strategies. And in this digital era, where data is readily available, leveraging exploratory data analytics can unlock valuable insights and help optimize event outcomes.
Introduction to Exploratory Data Analytics (EDA)
Exploratory Data Analytics (EDA) is an approach to analyzing data that aims to uncover patterns, trends, and insights by exploring the data visually and statistically. It involves examining and summarizing large datasets to identify relationships, outliers, and patterns that may not be evident at first glance.
EDA is different from traditional statistical analysis methods that require a pre-determined hypothesis to test. Instead, it allows for a more open-ended and flexible approach, making it ideal for post-event analysis when there is no specific hypothesis to test.
The goal of EDA is to understand the data, find meaningful patterns, and communicate insights effectively. It involves using various techniques such as data visualization, summary statistics, correlation analysis, and clustering to uncover hidden insights and tell a story with the data.
Data Collection and Preparation
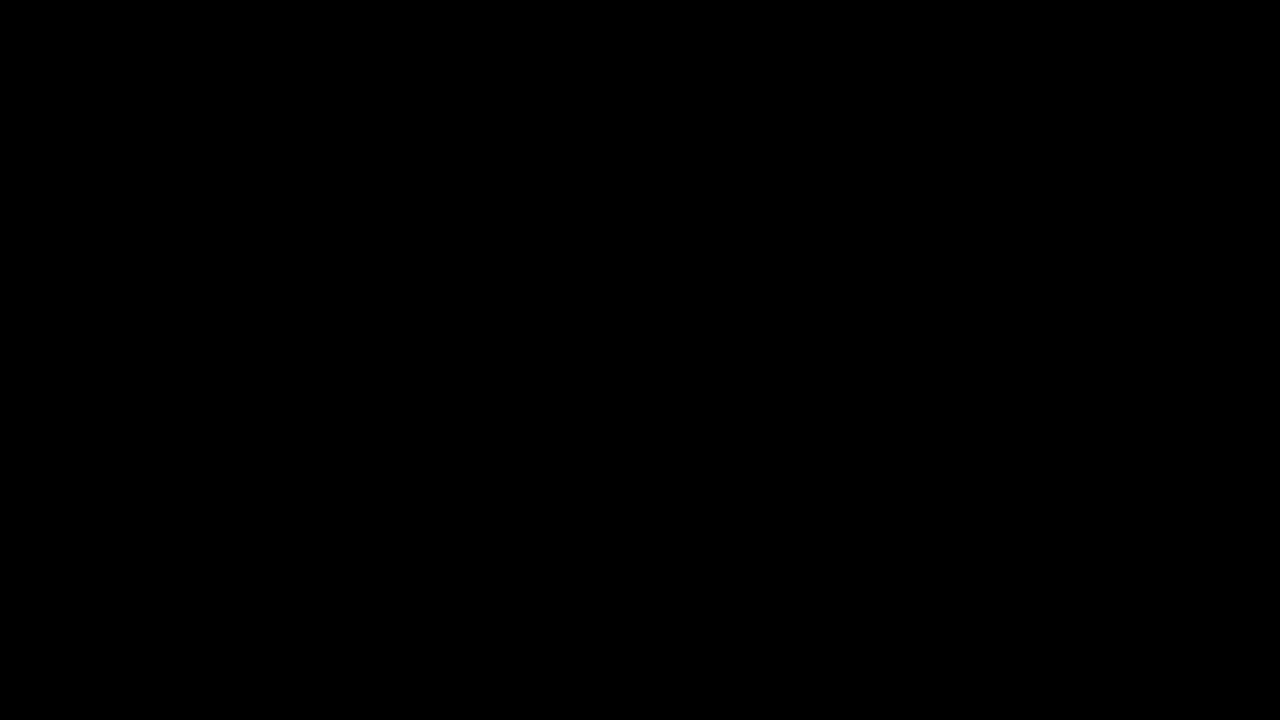
The first step in conducting EDA is to gather and prepare the data. This involves identifying the sources of data and collecting it in a structured format for analysis. Depending on the event, the data could come from various sources such as registration forms, surveys, social media, website analytics, and CRM systems.
Once the data is collected, it needs to be cleaned and prepared for analysis. This involves removing any irrelevant or duplicate data, handling missing values, and formatting the data in a consistent manner. In some cases, data from different sources may need to be merged to get a complete picture of the event.
Data preparation is a crucial step in EDA as it ensures that the analysis is accurate and meaningful. Garbage in, garbage out; if the data is not clean and well-organized, the insights drawn from it will not be reliable.
Identifying Key Metrics and KPIs
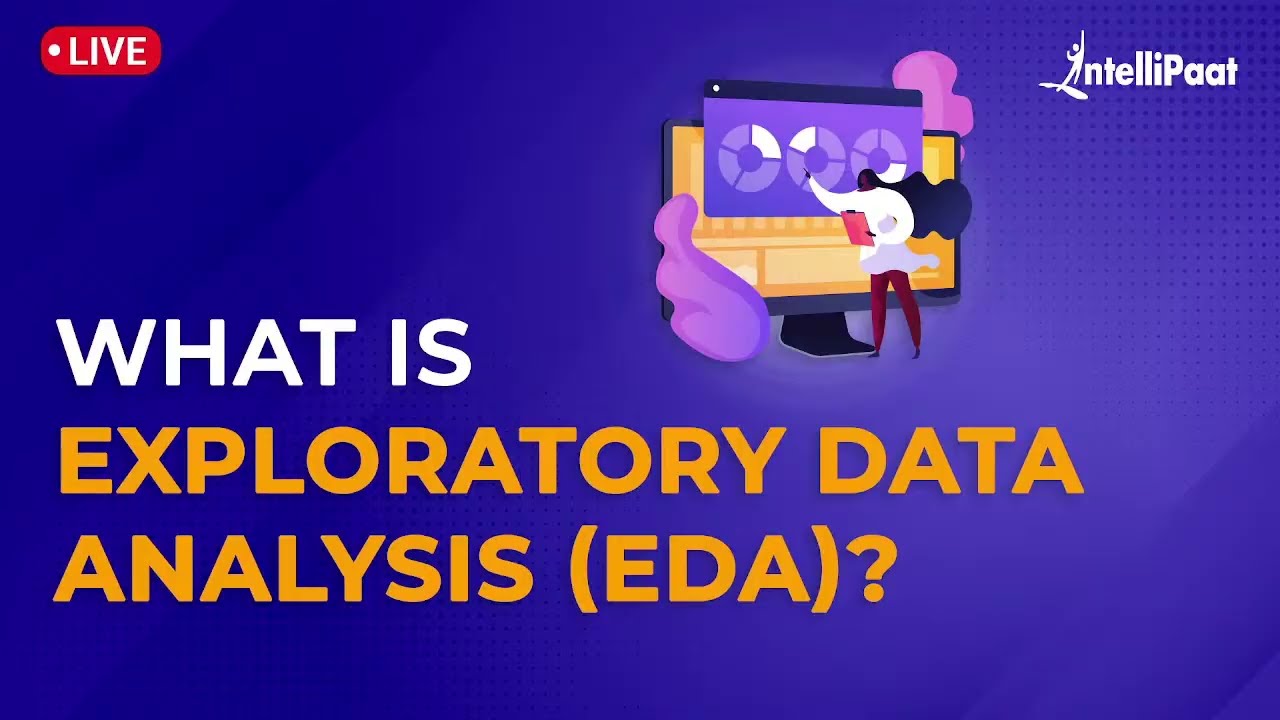
The next step in EDA is to identify the key metrics and KPIs (Key Performance Indicators) that will help evaluate the success of the event. These could vary based on the type and goals of the event, but some common metrics include:
- Attendance: The number of people who attended the event
- Registrations: The number of people who registered for the event
- Engagement: The level of interaction and engagement during the event (e.g., questions asked, polls answered, etc.)
- Social media mentions: The number of times the event was mentioned on social media platforms
- Website traffic: The number of visitors to the event’s website before, during, and after the event
- Leads generated: The number of potential customers or clients identified during the event
- Sales: The amount of revenue generated as a direct result of the event
Once the key metrics and KPIs are identified, they can be used as a benchmark to measure the success of the event and guide future strategies.
Visualizing Keyword Data
One of the most significant benefits of EDA is its ability to visualize complex data. Textual data, such as keywords and phrases, can be challenging to analyze and interpret manually. But with the help of data visualization techniques, we can spot patterns, trends, and relationships more easily.
For post-event analysis, keyword data can provide valuable insights into attendee interests, behavior, and sentiment. Keywords can be collected from various sources such as social media hashtags, event surveys, or website search terms.
One popular way to visualize keyword data is through word clouds. Word clouds are visual representations of the most frequently used words or phrases in a dataset, with the size of each word indicating its frequency. This type of visualization can quickly identify the most popular topics or themes associated with an event.
Another effective visualization technique is sentiment analysis. Sentiment analysis uses natural language processing (NLP) to analyze text data and determine the overall sentiment expressed. This can be helpful in evaluating the attendees’ reactions and feelings towards the event, speakers, or specific sessions.
Analyzing Trends and Patterns
Once the data is visualized, the next step in EDA is to identify trends and patterns. This involves exploring the data in more detail and looking for relationships between different variables. For example, we can analyze the correlation between event attendance and social media mentions or website traffic.
EDA also allows for the identification of outliers, which are data points that differ significantly from the rest of the data. Outliers could provide valuable insights into areas of improvement or success, and they should not be ignored in the analysis.
Clustering is another useful technique in EDA that can help identify patterns within the data. Clustering involves grouping similar data points together based on their characteristics. This can help segment the attendees into different groups with common interests or behaviors, providing valuable insights for targeted marketing and future events.
Actionable Insights and Recommendations
The final step in EDA is to communicate the insights derived from the data effectively. This involves summarizing the analysis and presenting it in a way that is easy to understand and act upon.
One way to do this is by creating an executive summary that highlights the key findings and recommendations. The use of visuals such as charts, tables, and graphs can make the analysis more engaging and easy to comprehend.
The insights drawn from EDA can be used to make data-driven decisions for future events. For example, if the analysis shows that social media mentions had a significant impact on event attendance, then increasing social media promotion for future events could be recommended. Or, if the sentiment analysis reveals negative feedback about a specific session or speaker, steps can be taken to improve those areas in the future.
Conclusion
In conclusion, exploratory data analytics is a valuable tool for post-event analysis. It allows for a deeper understanding of the event’s success, attendees’ behavior and interests, and areas for improvement. Through data collection, preparation, visualization, trend analysis, and presenting actionable insights, EDA can unlock valuable insights and help optimize future event outcomes.
In today’s competitive business landscape, leveraging data is crucial for staying ahead of the game. So the next time you host an event, remember to leverage EDA for a more comprehensive and data-driven approach to post-event analysis.